DOI:https://doi.org/10.34069/AI/2022.49.01.1
Applying Big Data technologies to counter cyber fraud
Применение технологий больших данных в противодействии кибермошеничеству
Abstract
By January 2021, the number of Internet users amounted to 4.7 billion, while the social media audience hit the 4.2 billion mark. Two-thirds of the world’s population use mobile phones daily. The average Internet user spends 42% of his time in the global network. These figures prove convincingly that the Internet has become an integral part of human life. However, man’s using the Internet involves increasingly the risk of cybercrime perpetrated against the user. The purpose of the research is to assess the potential of Big Data technologies to combat cyber fraud as a form of cybercrime. The study used the statistical data provided by the Prosecutor General’s Office of the Russian Federation and the publications in scientific journals. The methodological basis of the research is represented by a combination of general scientific and special scientific methods, with analysis, statistical method and systemic approach being the major tools. It was found in the course of the research that fraud constitutes the majority of crimes on the Internet. To counteract it, mobile operators and banks use anti-fraud techniques based on Big Data analysis. The paper provides an overview of services and programmes based on artificial intelligence and Big Data technologies, aimed at detecting and preventing telephone and internet fraud, used by law enforcement agencies in various countries. The paper concludes that Big Data has changed the vector of law enforcement activity from reactive to proactive.
Keywords: information, Big Data, cybercrime, cyber fraud, anti-fraud.
Аннотация
К январю 2021 г. число пользователей Интернета равнялось 4,7 млрд.чел., а аудитория социальных сетей перешагнула отметку в 4,2 млрд. чел. Две трети мирового населения ежедневно используют мобильные телефоны. В среднем пользователь Интернета проводит в глобальной сети 42% своего времени. Приведенные цифры убедительно доказывают, что Интернет стал неотъемлемой частью жизни человека. Однако это пребывание человека в Интернете все больше сопряжено опасностью совершения по отношению к пользователю киберпреступления. Цель исследования – сделать обзор возможностей применение технологий больших данных в противодействии кибермошеничеству, как одному из виду киберпреступлений. В ходе исследования использовались статические данные Генеральной прокуратуры Российской Федерации, Федерального бюро расследований США, публикации в научных изданиях. Методологическую основу составила совокупность общенаучных и частнонаучных методов, ведущими из которых стали анализ, статистический метод и системный подход. В результате исследования выявлено, что большую часть преступлений в Интернете составляют мошенничества. Для противодействия им операторы сотовой связи и банки применяют антифроды, в основе которых лежит анализ больших данных. Приводится обзор сервисов и программ, базирующихся на искусственном интеллекте и технологиях Big Data, нацеленных на выявление и предупреждение телефонного и интернет-мошенничества, использующихся правоохранительными органами различных стран. В работе делается заключение, что большие данные позволили сменить вектор деятельности правоохранительных органов с реактивного на проактивный.
Ключевые слова: информация, Big Data, киберпреступность, кибермошенничество, антифрод.
Introduction
In our days, information represents a vital resource for development of the society, a commodity for buying and selling, a currency and a weapon. It is collected by governmental bodies, intelligence agencies, industrial companies, mobile operators, market analysts, retailers, financial institutions, etc. It is used by all sorts of companies to form business models, optimise production, make forecasts, assess risks, seek efficient digital channels, etc. Information has become the backbone of economy and has rightfully gained the status of “new oil”.
Structured and unstructured amounts of data, as well as methods and tools for their processing are now called Big Data. The scope of Big Data application is huge, and the benefits of its use are undoubtedly great: owing to it, companies manage to attract customers’ attention, increase competitiveness and quality of service and resist cyber threats. Today, Big Data has an important impact on security; the results of Big Data analysis serve as a basis for decision-making (Wang & Wang, 2021).
The value of decision-making based on Big Data is especially growing in the field of countering cybercrime. This is much relevant since cybercrime is increasing significantly every year in many countries (Figures 1, 2).
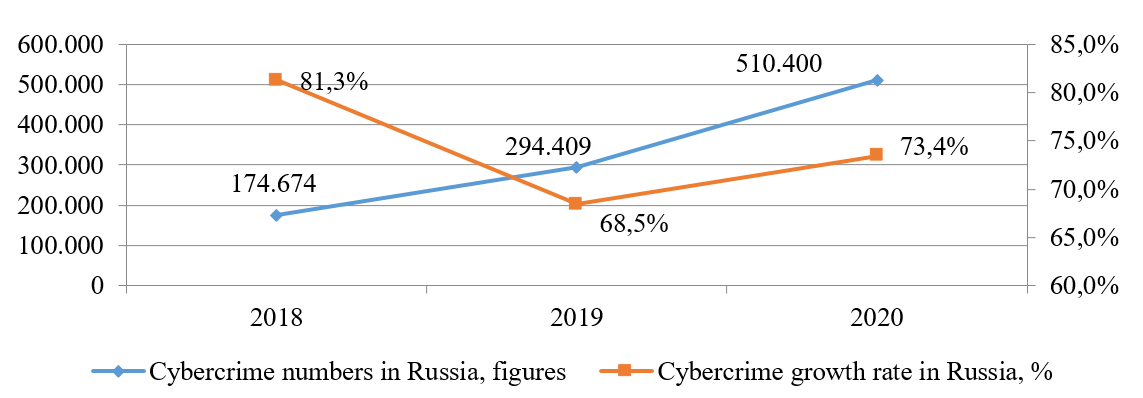
Figure 1. Number of registered cybercrime cases in Russia in 2018-2020.
Source: Prosecutor General's Office of the Russian Federation (2018, 2019, 2020)
As shown in Figure 1, cybercrime in Russia is growing rapidly – from 174,674 complaints in 2018 to 510,400 in 2020, or almost three times as much. The year-on-year increase in cybercrime is as follows: in 2018 – plus 81.3%; in 2019 – plus 68.5%; in 2020 – plus 73.4%. The same is specific of the USA. However, the growth rate in the USA is lower. In 2018 the number of registered cybercrime complaints was 351,937, while in 2020 this figure was already 791,790 – or 2.2 times as many. The complaints growth rate is demonstrated in the chart. The situation in Europe is similar (Kemp et al, 2020; Korsell, 2020).
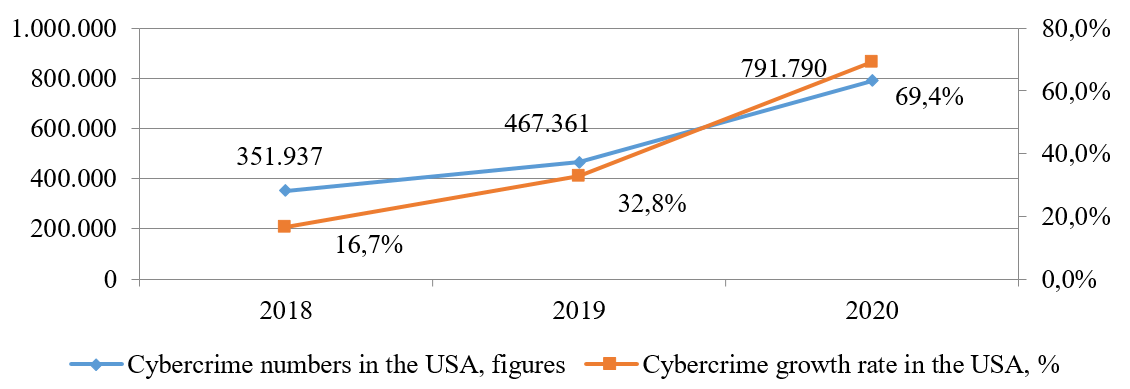
Figure 2. Number of registered cybercrime cases in the USA in 2018-2020.
Source: Federal Bureau of Investigation (2020)
Thus, the cybercrime sphere is a new challenge not only for individual countries, but also for humanity as a whole. The benefits of Big Data usage in security management prompted the authors of this paper to prepare an overview of the use of Big Data technologies in countering cyber fraud. The purpose of the research is to provide an overview of application of Big Data technologies in countering cyber fraud as one of the kinds of cybercrime. To achieve the set goal, the following objectives were to be handled: to provide an overview of the use of Big Data technologies to combat cyber fraud, to study Russia’s experience in this area, to propose possible ways of combating cyber fraud with the help of Big Data technologies.
Literature Review
Big Data can become an efficient tool in the fight against cybercrime, as increasingly noted by the researchers. Big Data can be used for searching and analysing the information that makes it possible to detect, investigate and prevent cybercrime (Singh & Bakar, 2019). Big Data technologies is a promising area for complex analysis of most diverse information. The use of this approach by financial institutions is designed to solve several important problems at once: to assess credit risks quickly and accurately, to prevent fraudulent actions, to increase sales (Al-Hashedi & Magalingam, 2021).
Big Data technologies make it possible to detect intrusion and anomalies, spam and spoofing, malware and ransomware, etc., which is crucial for cybersecurity (Alani, 2021). Big Data can be used to analyse certain patterns of behaviour, which in turn will help to prevent or prepare for a cyber attack and thus significantly reduce the scope of cybercrime (Apurva et al, 2017). A number of scholars have addressed the prospects and the potential of Big Data in the struggle against cybercrime (Najafabadi et al, 2015; Everett, 2015; Wall, 2018).
The importance of combating cybercrime today is inherent not only in the legal, financial and economic spheres of the society, but also in the political sphere: a number of countries, under the guise of punishing for cybercrime, exert political and economic pressure on other countries, including the one with the use of various coercive measures in the form of sanctions (Meliksetyan & Nusratullin, 2017; Nusratullin et al, 2021).Methodology
The purpose of the research is to evaluate the potential of Big Data technologies in countering cyber fraud as one of the kinds of cybercrime.
In the course of the work, the authors used the statistical data obtained from the Prosecutor General’s Office of the Russian Federation, the US Federal Bureau of Investigation, as well as publications in scientific journals and Internet sources. The combination of general and special scientific methods was used: abstracting and generalisation, which made it possible to systematise the facts and give their interpretation; logical conceptualisation method necessary for consistent presentation of the material; analysis and synthesis which secured the reliability of the conclusions; systemic approach designed to reveal interrelation between different phenomena; statistical method used for analysis of quantitative indicators.
This article attempts to analyse the experience of various companies’ using Big Data to combat cybercrime, as well as to consider the potential of Big Data technologies to be applied by law enforcement agencies.Results and discussion
In 2020, 25% of all crimes in Russia were committed using information and telecommunications technologies or those perpetuated in the sphere of computer information (Prosecutor General’s Office of the Russian Federation, 2020). The structure of such crimes is shown in Figure 3.
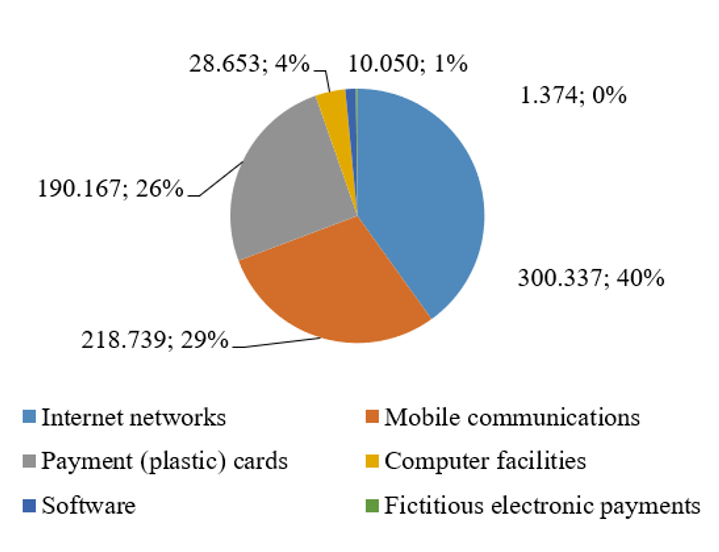
Figure 3. Number of registered crimes committed through the use or application of various information and telecommunication technologies, in figures and %
Source: Prosecutor General's Office of the Russian Federation (2020)
At present, the Internet fraud is represented in a variety of ways:
- phishing;
- scumming;
- spamming;
- carding;
- social engineering fraud;
- malicious software fraud.
Among the most efficient systems for countering cybercrime based on Big Data technologies are anti-fraud systems are systems for monitoring and preventing fraudulent transactions. Today they are actively used by mobile operators and the financial sector. The principle of antifraud operation is high-speed evaluation of a transaction and assigning a certain marker to it: green (the user is verified), yellow (medium level of suspicion), red (high level of suspicion) (Figure 4).
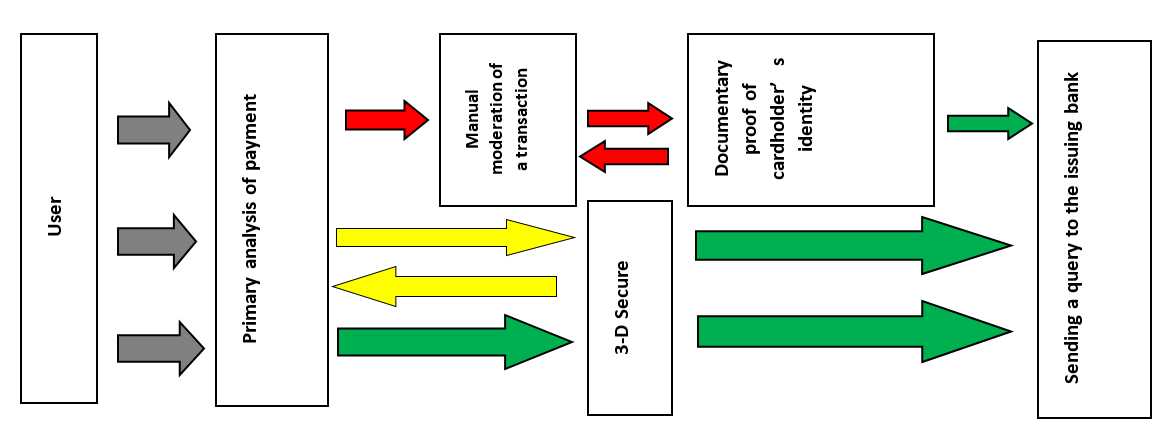
Figure 4. Scheme of antifraud systems operation.
Law enforcement agencies of various countries are now actively collaborating with IT companies which are developing technology solutions specifically for the former, intended to detect and prevent telephone and internet fraud, including illegal cryptocurrency transactions, hacker attacks, spamming, social engineering fraud, etc.
It is quite natural that cellular communication companies, banks and payment systems, along with law enforcement agencies, have proved to be at the forefront of the struggle against Internet fraud. It is these organisations that are now recognised as leaders in creating programmes and services designed to eliminate cyber threats. For instance, Chinese mobile operator China Mobile runs an application known as Tiandum (“Heavenly Shield”). It is based on Big Data analysis and machine learning technologies that enable the system to recognise the fraudsters’ characteristic phrases and intercept spam and calls from abusers. In order to train the system, the developers used the extensive database of fraud cases provided by police departments. Among the unquestionable strengths of the “Heavenly Shield” is its ability to identify user groups that are susceptible to risk of fraud most of all, to warn about possible attacks and, if fraud is suspected, to forward the numbers of potential victims to the police (Zhang & Williamson, 2021).
Banks and payment-system giants VISA, PayPal and MasterCard use Big Data successfully. Almost all of them have antifraud services – systems aimed at evaluating online transactions for content that is suspicious. Antifraud analyses a large number of parameters to identify potential fraudsters. Big Data help to create a profile of average payer; it is used as a basis for assigning a potential-fraud risk level to an operation. Users who do not leave digital footprints can arouse the system’s suspicion. If access is provided through social media accounts antifraud detects fake users (Larionova & Ryakhovsky, 2021).
Sberbank was the first Russian bank to use Big Data. In 2014 it developed a client identification system based on a photograph, using this technology along with the biometric system: comparison of a current photo with a photo from the database compiled by bank employees upon issuing a card. The result surpassed all expectations: cases of fraud dropped by a factor of 10. In addition, a service was launched jointly with the cellular operators Tele2 and MegaFon making it possible to identify telephone fraud in real time. Today similar services are also available in Tinkoff Bank and VTB. However, according to the experts, their significant drawback is that they are aimed at combating the consequences of telephone fraud, allowing to reduce risks, but do not exclude the root cause of it (Medvedeva & Vasin, 2019).
Several years ago, Big Data was adopted by VISA: using the open-source platform for reliable, scalable, distributed processing of large datasets through “Apache Hadoop” simple programming models, 500 aspects of a transaction are screened at once and 16 types of possible fraudulent schemes are verified (Etaiwi et al, 2017).
Big Data technologies have been used for quite a long time in law enforcement; their main purpose is to prevent possible crimes. For instance, some police stations in the USA and Europe have a proactive policing system that relies a lot less on responding to calls and increasingly more on patrolling areas known for high criminal activity. Searching for such areas is made automatically, which became possible owing to the development of Big Data intelligence systems that can collate relevant information and independently draw conclusions on increased criminal activity (Ovchinsky, 2017).
However, today artificial intelligence and Big Data technologies are used not only to analyse the crime situation in particular areas, cities, etc., but also for combating cyber fraud. For instance, in the Netherlands, “Bitfury Crystal” platform is used to analyse and detect suspicious cryptocurrency transactions; in the USA – “Chainalysis” cryptocurrency transaction analysis system along with “CipherTrace Scout” application – that allow to identify, track and document criminal transactions “in the field”, as well as to visualise them. In addition, the US Federal Bureau of Investigation actively uses “Mayhem”, a system designed to recognise the individual style of hackers and hacker groups, to detect attacks, to identify and pursue criminals up to locating them (De Vries, 2018; Gimenez-Aguilar et al, 2021).
Currently some major companies are creating technological solutions specifically for law enforcement agencies. Back in 2014, for instance, IBM presented its “Fraud detection system based on user-browser interaction analysis”. Its developers state that every user accesses the Internet from a particular device and shows a certain line of behaviour in various websites (including in online shops, banks, etc.), which pattern immediately changes if a bot or attacker gets involved. In this case, the system requires additional identification (Ali et al, 2019). This is specifically important with regard for the fact of broad automation of social engineering today: more and more frequently fraud is perpetrated by bots – programmes that are able to perform actions according to a certain algorithm, including engagement in dialogues in social media or forums. An advanced form of social engineering is exemplified by a situation when a person engaged in a conversation with a bot believes he/she is communicating with a human, since the programme is able to address a human user and maintain a conversation with him/her, producing answers which the human interlocutor considers to be natural. This system is able to meet the human interlocutor’s expectations, it is “socialised”, it maintains a dialogue within the framework accepted in the given community, is oriented by the developers towards inducing a person to perform certain actions – which is the criterion of its efficiency; this way it creates a real threat to the interlocutor’s cybersecurity (Sukhodolov & Bychkova, 2018).
The latest Big Data-based technologies aimed to combat phone and internet fraud are used by the law enforcement authorities of the Russian Federation as well. In particular, in 2021 the Russian Ministry of Internal Affairs is planning to launch a new “Antifraud” module. Its technical description specifies: “The mobile application of the Russian Ministry of Internal Affairs must have the functionality to check the local array of telephone numbers stored on the user’s mobile device against the array of telephone numbers contained in the local database management system of the mirroring server, with further addition of new telephone numbers or removal of irrelevant telephone numbers from the local array of telephone numbers stored on the user’s mobile device” (Sentsova et al, 2021). An undoubted advantage of the module is not only its ability to notify the user when he receives a call or a text message from a number registered earlier as a source of illegal action, with subsequent blocking, but also the availability of the so-called “white list” which is not subject to blocking and can be updated by the user on his own.
The experts note that the spread of cybercrime in general and cyber fraud in particular is forcing the law enforcement agencies to turn to latest technologies (including artificial intelligence and Big Data analysis) and to companies developing them. According to an opinion gaining popularity in Russia, there is a need to set up a cyber police force that will actively cooperate with highly skilled IT specialists – “Kaspersky Lab” and other organisations, which would make it possible to detect and prevent Internet crimes and reduce the timeframe for their detection and investigation (Shaporin et al, 2019).
Conclusion
The prospects of using Big Data in the struggle against cybercrime are great. Everyone realises this factor today: mobile operators, the financial sector, law enforcement agencies. The former, operating with huge amounts of information on their customers and having necessary resources, are actively creating various applications, systems and services aimed at eliminating cyber threats. The latter, often lacking the appropriate expertise, have to turn to IT companies for due developments. Owing to such cooperation, law enforcement agencies of various countries now have at their disposal programmes and modules aimed at detecting and, which is equally important, preventing crimes on the Internet, in particular as concerns illegal cryptocurrency transactions, spamming, fraud using social engineering technologies and malicious software, etc. It is the Big Data analysis that enables the police to be proactive rather than act in a reactive manner in the course of investigation of a crime, which means – to prevent it. This is particularly important in the case of cyber fraud, given the complexity of detection and investigation of such crimes, which, among other things, is conditioned by the transnational nature of cybercrime.