https://doi.org/10.34069/AI/2021.40.04.16
The practice of technological deception in videoconferencing systems for distance learning and ways to counter it
Практика Технологического Обмана в Системах Видеоконференций при Дистанционном Обучении и Способы Противостояния Ему
Abstract
This article raises the problem of high-tech deception using video conferencing means during distance learning, which is of increased relevance due to the digitalization of the educational process, with the growth of digital literacy of young people. The article presents some methods of fraud, including a relatively new technology that is very popular: Deepfake. The article details two popular tools for replacing faces, each of which provides step-by-step instructions on how to create, configure and apply in life. The organizational methods that are presented will help teachers detect even the most disguised forgery, including future voice spoofing, and stop any attempt at deception. At the end of the article, the system is described with the help of the technologies that used to combat deepfakes, and an assessment of the danger of existing tools is given. The objectives of this article are to raise public interest in the problem of face substitution and high-tech deception in general, to create grounds for discussing the need to switch to a remote format of events, to broaden the horizons of the reader and provide him with an area for further work and research.
Keywords
deception, deepfake, neural networks, machine learning, creating fake videos.
Аннотация
В данной статье поднимается проблема высокотехнологичного обмана при помощи средств видеоконференцсвязи во время дистанционного обучения, которая имеет повышенную актуальность в связи с цифровизацией образовательного процесса, с ростом цифровой грамотности молодежи. В статье приведены некоторые способы мошенничества, в том числе и относительно новая технология, пользующаяся большой популярностью – дипфейк. В статье подробно рассказывается о двух популярных инструментах по замене лиц, для каждого из которых приводится пошаговая инструкция по созданию, настройке и применению в жизни. Приводятся организационные методы, которые помогут преподавателям обнаружить даже самую замаскированную подделку, в том числе и будущую подмену голоса, и пресечь попытку обмана. В конце статьи рассказывается, с помощью каких технологий противостоят дипфейкам, и дается оценка опасности существующих инструментов. Целями данной статьи являются поднятие общественного интереса к проблеме замены лиц и высокотехнологичного обмана в целом, создание почвы для обсуждения необходимости перехода на дистанционный формат мероприятий, расширение кругозора читателя и предоставление ему области для дальнейших работ и исследований.
Ключевые слова
высокотехнологичный обман, дипфейк, нейронные сети, машинное обучение, создание поддельных видеороликов.
Introduction
More and more often, in the process of distance learning, videoconferencing technologies are used (Myownconference, 2019) both for conducting classes and for control activities, as well as conducting exams and even defending students' graduation papers. In the spring of 2020, in connection with the COVID-19 pandemic, the use of distance learning technologies increased significantly, which led to an increase in cases of deception of students and teachers during distance classes and exams.
In terms of deception on the part of teachers, one can note the practice of conducting automated webinars, when a pre-recorded training video is issued for online broadcasting, and artificial intelligence technologies answer questions from listeners in the webinar chat in relation to video content, or include the necessary part of the recording depending on the general mood of the audience. However, this phenomenon is more characteristic of brief events and is not yet systemic in distance learning, therefore it will not be considered in this work.
The most widespread technologies and technological methods of deception by students in the process of conducting distance video lessons and during control activities. It is they who are the object of consideration in this work.
Risks of Academic Deception
Researchers at the Institute of Education of the Higher School of Economics (HSE, 2020) note that to describe the phenomenon of academic deception, one can use two theories explaining dishonest behavior: the general theory of crime (Gottfredson & Hirschi, 1990), in which deception arises as a lack of impulse control when trying to immediately realize desires, and the theory of cognitive unloading (Simon, 1982), when the student is inclined to reduce cognitive stress for the purpose of which he takes actions to deceive. Researchers consider proctoring technology as the main way to protect against academic fraud, allowing to conclude whether the fact was fraudulent or not.
From the perspective of the theory of crime, one of the important points is the significance of the results of control activities (including attendance in the classroom) for students. Based on this, in activities with high stakes for students, academic cheating will be more likely to take place. So, if attendance indicators are included in the rate, the likelihood of deception in the process of video lectures and webinars will significantly increase. Based on this, it is more rational to organize the educational process in which the rate of control activities is reduced: for example, instead of a large final test or exam, the organization of a series of events that contribute to the final grade of the course. In addition, monitoring of attendance at online classes in higher education should be carried out without including it in the course rating.
Currently, video conferencing technologies are widely used for conducting classes, in particular MS Teams and Zoom, in which the possibility of parallel display of several students in the course of conducting classes is implemented. However, if you do not organize active feedback with students in the process of such classes, then even if there are windows with interlocutors, one cannot be sure of their real presence in the class. So, in practice, when conducting a lecture in mathematics in an online format for 120 students, based on the results of random control by the teacher, it turned out that 15 students were actually involved in the work and the rest had simply turned on their devices and continued to do other activities. In addition, when organizing classes for an audience of more than 12 people, it is very difficult to control the emotional mood of the audience.
Technologies that make it possible to “seat” students in a virtual classroom are emerging (Figure 1). For example, MS Teams technology can accommodate up to 49 participants. Nevertheless, further we will consider technologies of deception of this solution and Zoom using easy-to-install software. Therefore, the second recommendation is to reduce the cognitive stress of students in the course of such classes by the dosed presentation of educational material.
One of the problems identified in practice is the reluctance of students to turn on their video cameras even if they are available and the possibility of using a virtual background. The experience of conducting classes at Moscow Aviation Institute (MAI) in the spring of 2020 showed that no more than 10% of students are ready to participate in events with a webcam turned on, about 25% refer to the lack of equipment, 35% of those with equipment go out only in voice mode, and another 30% include video broadcasting only at the request of the teacher (according to the analysis of control activities of the technical video conferencing service based on BigBlueButton and Scalelite). Thus, at best, the virtual audience will be filled with only half of the participants in the online lesson.
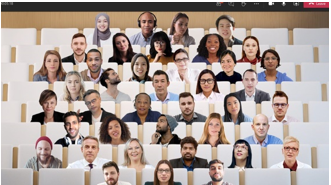
Figure 1. Example of placement of video conference participants on a virtual background imitating a classroom.
Technologically, the risks of deception can reduce proctoring systems, but they also have their drawbacks. At MAI, using the Examus proctoring system, exams were held for applicants under the pre-professional exam program. This exam adds 5 points for admission to the university (out of 310 possible). Since this is an additional opportunity for applicants, this exam can be attributed to an event with high stakes, which is why a special control system was used. According to the results of the exam, 110 out of 256 subjects passed it. At the same time, the participants undertook various technologies to deceive the proctoring system. Fortunately, none of those who used the technology of deception scored more than a passing score and there was no need to cancel the exam results. Some of the technologies and behaviors used will be described below.
Proctoring systems and software for their deception are constantly being improved. Therefore, below we will consider promising technologies of deception and possible ways of protecting against them.
Theoretical Basis
Technologies and strategies of academic deception
We will consider a number of technologies and strategies of academic deception that we have encountered in practice. They can be conditionally divided into the following two groups:
- imitation of activity and/or presence during online lessons;
- academic deception in tests and exams using video conferencing.
A common scenario of deception in the first group is associated with lectures, in which attendance was monitored. Depending on the attendance control technology, the following methods of academic deception were identified:
- transfer of logins and passwords from the training system or video conferencing service to another person to ensure the entry of all participants and their simultaneous presence in the conference chats;
- connection of virtual devices to simulate the presence in the lesson with video, in particular, the widespread use of pre-recorded virtual backgrounds from the student's video in a looping mode (usually about 3-5 minutes);
- primary entrance to the lesson and participation in the attendance control procedure from any device (more than half from a mobile phone), followed by leaving the workplace or transferring the conference on the device to the background.
Based on the analysis of these methods, the following measures were proposed:
- changing passwords with the transition to a single authorization within MAI and the addition of services available under a single password to the structure of services containing confidential data and allowing to perform legally significant actions, which significantly reduced the risks of transferring passwords between students;
- recommendations for teachers on periodic feedback sessions in the question and answer format (Q&A) with a frequency of at least 15-20 minutes and conducting a dialogue with the audience during the lecture through chats.
The use of online services customized by an educational organization is worthy of separate consideration. For example, when using a university application in the App Store or Play Market, you need to be prepared for the fact that students can artificially lower the rating of the application and bring down the system as a whole (lowering the rating will lead to the removal of the application). An example is the DingTalk application for Chinese schoolchildren. After schoolchildren figured out that the app would be removed from the App Store if a sufficient number of users give the application one star, the app's rating dropped from 4.9 to 1.4 overnight. MAI uses an application for the moodle system and so far, these trends have not been observed.
The same applies to the infrastructure of the university, but this is a topic for a separate study, since it is possible to bring down the learning management system of an educational organization by the simultaneous entrance of all students. Many universities have faced the unavailability of their systems due to high loads.
Academic deception during control activities is mainly associated with the tasks of bypassing proctoring systems and / or imitating behavior in a video conferencing system. These are the typical methods of deception that we have encountered in practice:
- simulated connection breakdown is the most common deception strategy, due to the fact that many regulations provide a clause that a student has the ability to restore the connection within 5-10 minutes in case of technical difficulties (to counteract this strategy, it is required to control the connections, video signal and computer peripherals, which at this stage are not implemented in many proctoring systems);
- connection of a second monitor, where prompts are displayed or a search engine is used to search for answers (for counteraction in control activities, it is recommended to give tasks that do not have ready-made answers, but this is not always applicable). For example, to solve tasks on math, students used the Derive system. Some proctoring systems have a check for the presence of a second monitor, but this is easily circumvented by correctly placing the camera between two monitors and simulating a large screen);
- connection of a second computer, similar to that discussed above, allows you to bypass the protection of proctoring systems if the second computer is correctly placed (there are solutions for proctoring systems with a mobile phone involved, but there are ways to bypass this solution); connection of a second computer, similar to that discussed above, allows to bypass the protection of proctoring systems if the second computer is correctly placed (there are solutions for proctoring systems with a mobile phone involved, but there are ways to bypass this solution as well);
- virtual camera is a fairly common strategy when conducting certification activities that allows you to turn on looped video and sound of work (there are solutions that allow you to compare sound and data input from the keyboard and mouse, but these are quite expensive, proctoring methods);
- substitution of an attested at the place of passing the exam is an even rarer strategy in which a student who is similar to the one being attested is selected, who has the necessary knowledge and who passes the exam from his personal computer with the transfer of identity documents (in practice, it was used only once, it is possible to detect it by using intelligent services of identification of the person and a number of technologies that will be discussed below).
In addition to technical measures, simple organizational measures can also be used. So, for high-stakes replacements, it is better to terminate the procedure and complete the attempt in case of technical problems, without giving time to reconnect. This can be interpreted by students as unjustified requirements; however, in practice, this can significantly reduce the number of technical problems, because the students in the event of such a requirement are tested in more detail the equipment and the Internet connection.
How would a service that offers to “substitute” a student for an exam would look like?
Supposing that at the moment there is a service that will allow an expert in a particular field to act as a student taking an online exam, we will try to give an approximate algorithm of work:
- 7-10 days before the exam, the student contacts this company for help. The technician requests the following high-quality materials from the customer:
- 3-5-minute video, in which the student will make various slow movements of his head up, down, left, right and in a circle, utter 10 difficult phrases, which will contain the maximum number of different letters and letter bundles, often and rarely used in speech, moves his eyes in all projections and expresses several emotions - fear, fear, surprise, joy, anger, anger and so on;
- 30-minute audio track on which the student reads a complex scientific article and literary work in a clear voice of different loudness;
- technician starts processing the material: cuts frames in the video, highlights the face, forms masks and trains the neural network for a week. A chalk-spectrogram is formed from the audio, considering various parameters such as tonality, timbre, volume. At the end of the work, casts of the face and voice are obtained, which can be used on the day of the exam;
- on the day of the exam, the student provides information to access the training system and a background image. An expert in the subject of the exam enters the meeting. The background is projected onto the chroma key behind him. The expert connects to the examination system, launches the face and voice models, and passes the exam for the student. The shots are very accurate, and an inexperienced teacher will not be able to find out that he has been deceived.
This service would be very popular, but it would require huge financial investments: the purchase of powerful equipment (servers, video cards, processors, etc.), rent of premises, payment for the work of experts, electricity bills, etc. There would be dozens of students' requests per day, each of which would need help. It would be reasonable to say that the price for which a student could use the service would be very high, but such an investment would be justified.
Where did Deepfake come from?
Convolutional codes and neural, convolutional and generative adversarial networks (GAN) and machine learning: these are the things without which the deepfake would not have been created.
Convolutional codes: they can correct errors and use continuous or sequential processing of information in short chunks (blocks). Convolutional codes have memory because they are characters at the output of the encoder depend not only on the characters at the input, but also on the previous characters passed through the encoder. A convolutional encoder is a sequential machine or finite state machine. The state of the encoder is determined by the contents of its memory. The main difference between convolutional and block codes is that the n output code symbols depend not only on the current information block of length k bits, but also on m previous information blocks. Convolutional coding can be implemented using a linear chain with k inputs, n outputs and a memory of dimension m. Typically, n and k are small integers, where n>k. At the same time, to provide the necessary noise immunity, the dimension of the memory m must be significantly greater than the parameters n and k (Lobachevsky, 2020).
Neural networks: it is a technology used in science and engineering. With their help, programs are improved and whole systems are created that can automate, speed up and help a person in their work. The main goal is to teach the system to independently make decisions in difficult situations, as a person does. The first neural networks appeared at the end of the 1940s, but they began to be fully used only in the eighties, when computers received sufficient computing power. Over the next 20 years, the power of computers grew so much that in 2000, research scientists were able to apply neural networks in many areas. Programs for speech recognition, visual imitation, cognitive perception of information appeared. Neural networks, machine learning, robotics and computerization have become part of something called “artificial intelligence” (Future2day, 2020).
Convolutional neural networks: this is one of the most popular types of networks, often used to recognize certain information in photos and videos, language processing and recommendation systems. Their main characteristics are:
- excellent scalability: they carry out image recognition of any resolution;
- use of volumetric three-dimensional neurons: inside a layer, neurons are connected by a small field called “receptive layer”;
- use of a mechanism of spatial localization: neighboring layers of neurons are connected by such mechanism, due to which the operation of nonlinear filters the coverage of an increasing number of pixels of the graphic image (Future2day, 2020).
GAN: they are an architecture consisting of a generator and a discriminator configured to work against each other. They are the basis of all Deepfake technologies. It was created in 2014 by Ian Goodfellow, a student at the University of Montreal. Discriminatory algorithms try to classify the input, considering the peculiarities of the data obtained and then trying to determine the category to which they belong. Generative algorithms do the opposite: instead of predicting a category from the available images, they try to match images to that category. One neural network, the generator, generates new data instances; and the other, the discriminator, evaluates them for authenticity and decides whether each data instance that it considers is a training data set or not. At this time, the generator creates new images, which it passes to the discriminator. He does this with the expectation that they will be accepted as genuine, although they are fake. The purpose of the generator is to generate images that can confuse the discriminator. The purpose of the discriminator is to determine if the image is genuine (Borshigov, 2018).
Machine learning: it is the process of adjusting the parameters of a neural network to obtain the desired result at the output for various input parameters. The input signal cannot be changed, the adder performs the function of summation and it will not work to change something in it or remove it from the system, since it will cease to be a neural network. There is only one thing left: to use coefficients or correlated functions and apply them on the weights of links (Future2day, 2020).
What is Deepfake?
Deepfake is a technology for synthesizing images and sound using artificial intelligence, with the help of which it is possible to simulate human speech and behavior and simulate a picture that can make a person do what he has never done and say what he never said. The first developments were created back in the nineties, but only specialists in the film industry could use Deepfake. Years later, in 2014, the American researcher Ian Goodfellow pioneered the Deepfake technology, based on GAN designed by him and his colleagues. For several years, only a close circle of people had access to the development, until in 2017 the technology appeared in the public domain. Initially, the works created with the help of the new program had no serious connotation: the first deepfake video is the superimposed face of Gal Gadot on a random actress in a pornographic video. Then it started to appear in various applications such as Snapchat, which “drew” different masks, decorative elements and accessories, other faces, etc. to the original human face, or the more dangerous FakeApp application, which made it possible to completely replace the face without any trace. The technology of imposing various effects appeared in such large social networks as Instagram and Vkontakte, and videos began to appear on YouTube, where now it is possible to see how an actor plays in a film in which he never starred (Panasenko, 2020).
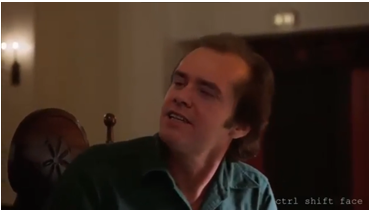
Figure 2. Jim Carrey appears in a The Shining scene instead of Jack Nicholson (Baikinova, 2020)
But if initially Deepfake was conceived as an auxiliary application that could facilitate work in some industries or save time, then later this technology began to be used for personal gain. In 2018, provocative videos with well-known and influential politicians appeared on the network, undermining their reputation and destroying the trust of random people, and in 2019 a major fraudulent operation was carried out: an attacker using another related technology, DeepVoice, forged the voice of the company boss and, having deceived the managers, withdrew about 243 thousand dollars from the company's accounts. Some fake videos are a means of blackmail and cyberbullying, while others show people using this technology to impersonate another individual during an online conference or exam. The amount of such content is inexorably growing every year: to create such videos, it is enough to familiarize yourself with the technology for creating deepfakes, rent a cloud server (if there is lack of suitable computer equipment), upload the initial data there and get the finished result in a few days. We will take a closer look at the process of creating fake roles and find out what this technology is based on (Pandasecurity, 2019).
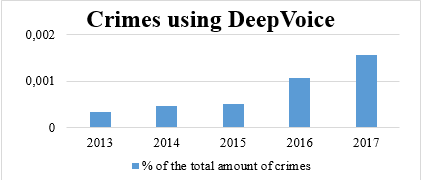
Figure 3. Statistics about cybercrimes using DeepVoice (Pindrop, 2018)
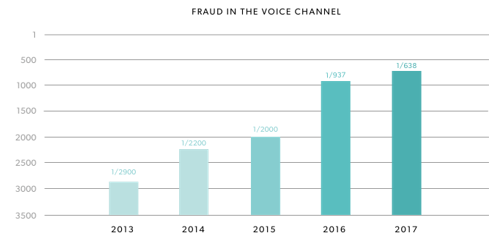
Figure 4. Statistics about fraud in the voice channel (Pindrop, 2018)
Methodology
Manipulation of faces occurs using two modifications: personality modification (replacing one face with another (DeepFake and Face-Swap work with this method) and facial expression modification (transferring the features of one face to another, which is the basis of Face2Face's work). There is a large number of applications that can replace your face with someone else's: FakeApp, Face Swap, Doublicat, Snapchat's Lens Studio, etc. We will briefly consider each of the above (Kumar, 2019):
- Lens Studio is an augmented reality application that overlays one image on the standard control points of another. This application is not suitable for creating full-blown fakes;
- Face Swap is focused on working with static images and is not very efficient. This application is more based on simple graphical work than on complex neural networks that require long training;
- Doublicat is already more like a deepfake application, but at the moment this product works effectively only with standard and already trained models, while the quality of user experience leaves a lot to be desired;
- FakeApp is a complete analogue of the DeepFaceLab application, which will be discussed later. These programs differ only in their designs. As their replacement algorithm is similar and the end user himself can choose what is convenient for him.
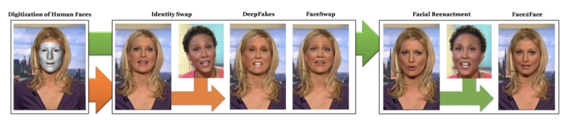
Figure 5. Operating principle of modifications (Rossler et al., 2019)
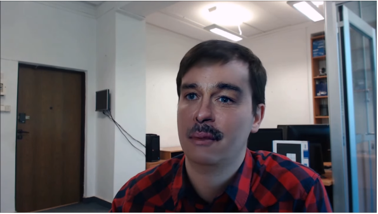
Figure 6. An example of how FaceSwap works. The frame shows irregular highlights, ghost zones and a curved blend in the lower face. Therefore, we can say that the effectiveness of this application is not close to ideal (Github, 2020a)
We will talk about two of the most popular applications today: DeepFaceLab from iperov, which is designed to create videos offline without time limits; and Avatarify (Face2Face technology) from alievk, which is best suited for real-time fakes.
The concept of DeepFaceLab is to transfer the face of person A from one video to the face of person B from another. The work will require thousands of frames, which will depict faces A and B separately from each other, a deep learning convolutional neural network and some time to train it.
Initial training is performed first. The encoder takes each face image and then decodes them, restoring the original frame. The neural network is trained to quickly identify a face at key points (eyes, lips, cheeks, eyebrows, nose, etc.). At this stage, each person has their own separate decoder.
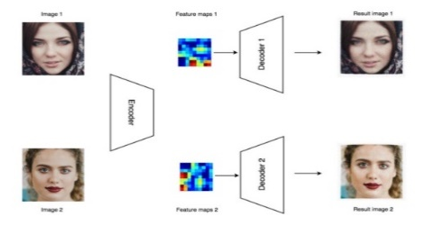
Figure 7. A neural network learns to quickly encode and decode a face using key points.
At the next stage, the program collects a new video. The neural network selects face A, encodes it, but the face decoder B is already used for decoding. At the end of the stage, the new generated face is merged with the original frame.
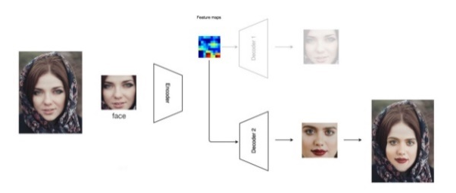
Figure 8. Face B is replaced by Face A at key points.
The encoder consists of 5 convolutional layers, each of which defines key points. These layers are followed by another two dense layers, followed by the decoder's convolutional layers, which restore the original image by using the previously selected key points. Intuitively, the decoder detects the angle of the face and its expression, skin tone, lighting and other important information. Thus, the decoder reconstructs the frame by drawing face B in the context of face A. This system of convolutional layers is called “autoencoder” and is the most important link in the process of creating a deepfake.
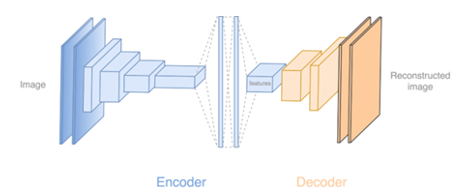
Figure 9. How autoencoder Works.
In order for the face to be decoded without border discrepancies, a new face mask is created and used in the final frame. Furthermore, to avoid artifacts (wrong eyes, mouth, skin tone in some places, etc.), a Gaussian filter is applied, which scatters the edges of the mask, allowing to make a better overlay, as well as several manual settings that will allow to change the size of the mask created by the neural network. However, even the highest quality masks may have ghostly edges or double chins as a result of the fusion.
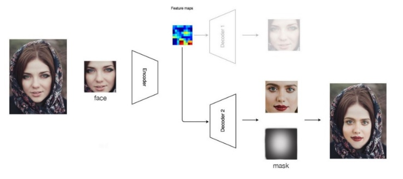
Figure 10. Replacement of face B with face A with a mask.
In order to improve the result of merging two faces, it is necessary to use a new technology: a mask that links both the initial image and the finished one. This technology is used in GAN.
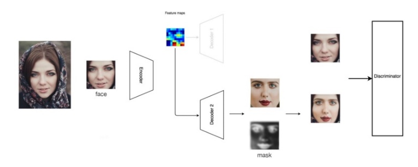
Figure 11. How GAN work.
If the original frame hits the discriminator, the network learns to better identify the real image. If the created frame gets into the discriminator, the autoencoder learns to create a more believable image. This competition continues until the created pictures become difficult to distinguish from the real ones.
In addition to training the neural network, GAN improve the mask. They become clear, sharp and accurate, while the autoencoder learns to create even incomplete masks in those moments when the face is covered by hands, hair or something else. This method is very long and laborious, but it has the highest effect.
Results
Creation of a deepfake video
Now we will take a look at the process of creating deepfakes from a practical point of view. All utilities can be found and downloaded on the official website of the developer, the link to which is indicated at the end of the article. To work with this, two video files are required: one with the person to be replaced and another with the person-substitute (video donor). For an effective result, the video donor should be long (2-3 minutes), since more material will produce a more accurate “cast” of the new face. The first video will be named data_dst (destination is where it will be superimposed), and the second (donor), data_src (source) (Github, 2020b).
Then the workspace should be prepared, clearing it of all unnecessary things by running script 1. We need to load the source videos into the workspace folder and run scripts 2 and 3, which will cut each file into separate frames. After storyboarding, we should run scripts 4 and 5, which will determine all faces on each video fragment, and then by using scripts 4.1 and 5.1 we will look at the final result and manually delete the “wrong” or unnecessary frames. Scripts 4 and 5 have several modes:
- full_face and whole_face modes. For the best result, we choose the second option, but there is no big difference both of them;
- modes MANUAL, S3FD and an option that combines both. The most accurate option is S3FD. MANUAL is used to manually retrieve already extracted faces in case of errors at this stage. The combined option allows to manually specify the contours of the face in frames where it has not been detected. At the same time, at the end of file extraction, a window for manual contour correction is opened;
- MANUAL + RE-EXTRACT mode, which is a duplicate of the MANUAL mode but with one difference: after the initial extraction, the faces will be extracted again. This mode is designed to train the neural network and improve its performance in the future.
It is important to remember that script modes 4 and 5 must coincide, otherwise the program will generate an error (Proglib, 2020).
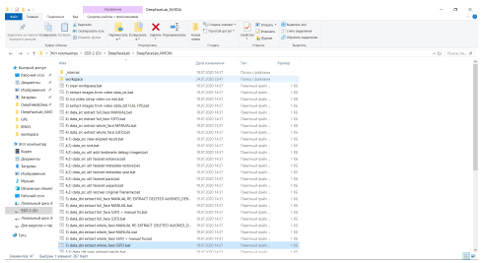
Figure 12. List of all Scripts available in the program DeepFaceLab (part 1)
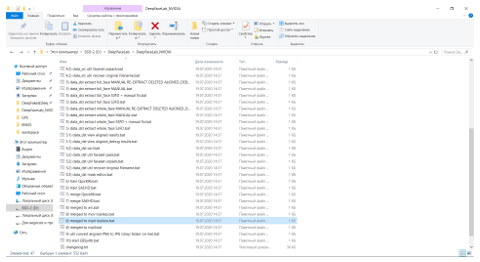
Figure 13. List of all Scripts available in the program DeepFaceLab (part 2)
For better orientation in the extracted faces, the program offers various sorting options. It is also possible to pack and unpack frames if the user plans to continue working on another device.
The script 5.3 is a key one. At this stage, the area of the final substitution is marked, and the neural network itself will select the optimal replacement area. After automatic work, the mask may not capture the forehead, but there is nothing wrong with that, since further in the place of imposition there will be a smooth and imperceptible transition from the old face to the new one. If desired, it is possible to expand the replacement area by manually marking it. Here the program also makes it easier for the user to stabilize the position of the head relative to the center of the screen: it is enough to create a mask only for the first frame and correct it in places with strong deviations.
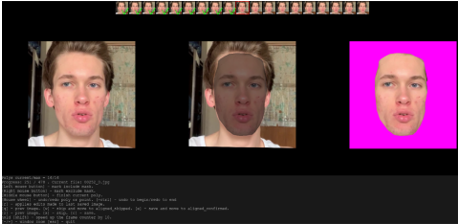
Figure 14. Visual representation of the 5.3 script for creating final masks.
At the next step, training of the neural network begins and the discriminator is turned on. This stage is crucial, but also the longest one: it takes at least 6-8 hours of non-stop training to get acceptable results. To get started, the script 6 should be run. It has two codec options:
- Quick96 codec, which is designed for weak video cards (up to 2 GB VRAM) or for fast work on powerful devices. 2-4 workouts are performed every second. However, the quality of the final picture will be very poor. The training starts immediately;
- SAEHD codec, which is designed for video cards with a VRAM of 6 GB or more. This codec will be slow and resource intensive, but the result will be a perfect face replacement. For training, you need to specify additional parameters.
First, we should create a model (or work with an already created one) and select a platform for work (the processor or one of the video cards, if you have several of them, the default platform is the main video card). If you select the SAEHD codec, additional options are offered. Initially, each item has default values, but it is recommended to check the Face Type tabs (should be “wf” if whole_face is selected in script 4 and 5) and Eyes Priority (if the value is “y”, the new character will blink in the final video and move your eyes like the original, but in this case, the process will take longer).
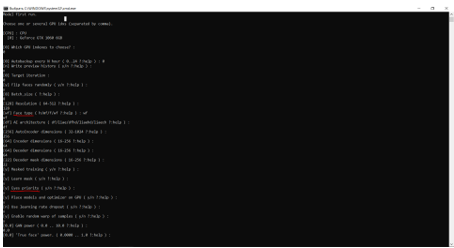
Figure 15. Neural network training parameters.
After setting all the parameters, we start the process. The minimum number of iterations is 30.000-40.000, the optimal one is 100.000. The iteration window will open, where you can find faces, their masks and the result of merging, as well as yellow and blue lines. One of them is responsible for recognizing the original by the discriminator, and the second is responsible for recognizing the copy. If these lines coincide, then the neural network begins to get confused: we can assume that the minimum optimal variant has been reached and the process can be completed.
The next item is script 7, “merge”. It also has two modifications, depending on the previously selected codec, we launch the desired one. On each fragment, we adjust the necessary mask parameters (sharpness, blur, color) for greater similarity.
The final step is frame-by-frame assembly of the video. We select any of the scripts 8, wait for the end of the process, go to the workspace folder and take the result. This completes the process of creating a deepfake.
The above technology could work in real time, but everything here depends on the power of computing technology and the initial data, which would require much more for such a purpose. But there are other applications based on this technology. The FaceIT_live program from alew3 replaces the face in real time according to a pre-trained model. The principle is the same: several thousand photos with the donor face in different positions are loaded, masks are formed, the model is trained (it takes about 48 hours). The program captures a frame from the webcam, runs it through the autoencoder and convolutional layers, and returns a new image. But in applications that work immediately, there is a high probability of the appearance of flaws, and the chance of revealing a forgery using various organizational methods is significantly higher (Github, 2020c).
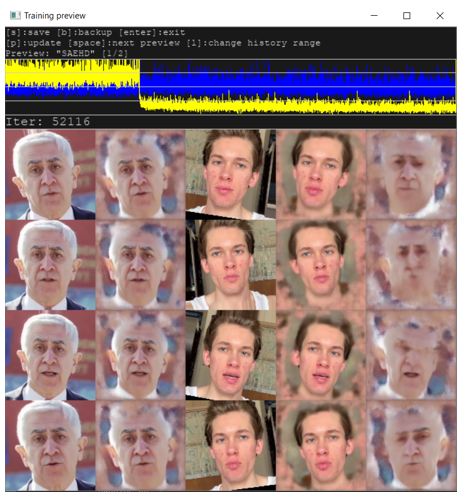
Figure 16. Visual representation of the iterations during the process of creating a deepfake.
Real-time face and voice replacement
To replace a face in real time using the simplified Face2Face technology, the application Avatarify has been created, which can be downloaded from the developer's official website. In order to work with it, a webcam is needed together with the OBS-Studio application, which relays the image to other video communication applications. After starting the program, we should center the position of the head in such a way that it coincides as much as possible with the position of the original face for better snapping of key points. The program is useful if you need to substitute a person at a conference or at other events organized using video conferencing (Github, 2020d).
The voice can be replaced using DeepVoice technology based on neural networks and machine learning. For effective work, it will take about 1.000 hours of conversation with the voice and some time to create an ideal chalk-spectrogram: a value that includes various parameters of the voice. In real time, voice forgery, as well as face forgery, is currently impossible due to technical difficulties and insufficient research in this area (Kireev, 2019).
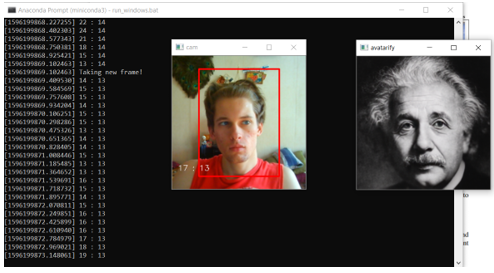
Figure 17. Interface of the program Avatarify.
Discussion
We will suppose that a professor is conducting an exam online. All students are required to enter the exam environment and connect to the webcam broadcast so that the examiner can see their faces. At the same time, the teacher knows about the existence of face replacement technology, and therefore, during the exam, he can reveal the suspect in an attempt to unfairly pass the exam in the following ways:
- to begin with, the teacher should superficially examine the face of the student. If there are crooked edges, dark spots, light and color inaccuracies, ghostly edges, etc., then we can confidently say that the student is not who he claims to be;
- the next step is to check the movement of their eyes. The professor should ask a student to move his eyes in different directions, as well as blink on command. Not all neural networks are precisely tuned for eye movement, so often this area of the face can give out a deepfake;
- then, the teacher should focus on facial expressions. The student should be asked to express surprise, fear or any other emotion, or say any difficult letters or words that the examiner has compiled beforehand. There are small details in this test that are very difficult to convey using face replacements;
- if the previous checks showed nothing, then the teacher should ask the student to make a couple of sharp movements with his head, to move it within the frame capture area, to move his hand, textbook or other object in front of his face, to get up from his workplace and then sit down, etc. The mask is very sensitive to non-algorithm movements, and a sudden change in the scene or an incomplete area of the face can cause a half-second failure, after which its operation can return to normal. However, this half a second is enough for the examiner to give the student a negative mark;
- the student who is taking the exam can go for a trick and artificially underestimate the quality of the transmitted image, thereby the difference between the original face and the applied mask will be practically invisible. In this case, the teacher should ask the student to improve the quality of the picture, and if this is not possible, then move the video camera to another place or ask him to use another camera and show the recording at the end of the exam. The latter option is a guaranteed way to detect a face replacement during an exam, since there is no technology that can replace a person's face in real life;
- we also have the technical method: ask the student to turn on a desktop demonstration, open the task manager and complete some suspicious processes or check the loaded video card and processor. The creation of the most plausible replacement of the face requires a large amount of RAM, and the processor cores and video chip would be heavily loaded, which will certainly cause suspicion;
- voice replacement is also possible in the future. To test this deception, we asked a student to utter a few tongue twisters, expressively read a poem, shout, cough, or sneeze.
Conclusions
To date, video conferencing systems are the most comfortable way of communication. However, this environment is highly susceptible to deceptions using high technologies: Deepfake and DeepVoice allow one person to be passed off as another so that no one will suspect anything. Sometimes it seems that it is impossible trust anyone on the Internet, and it is time to give up video communication and hold events in real time. However, technology, although it is developing rapidly, is still unable to deceive a person without flaws, and there are organizational moments and tricks that allow to reveal the deception.
Also, there is no need to worry about the fake videos created offline. In mid-June 2020, Facebook and Microsoft summed up the results of the Deepfake Detection Challenge, during which developers identified fake material (Westerlund, 2019). Based on the results of work on simple videos, the new programs were able to determine the fake with an accuracy of 82% and on complex ones (with filters and captions), with a 65% accuracy. Given the high quality of the prepared material, new developments have shown very good results, capturing the smallest and most inconspicuous details that give out a fake (Purwins et al., 2019). This means that even the most advanced technologies designed to deceive ordinary people cannot yet do it in full, and the fight against high-tech deception will be waged until complete victory.